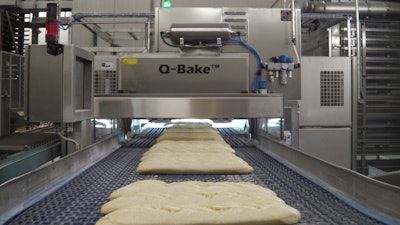
Artificial intelligence (AI) in food processing is no longer a grand idea – it is already making significant impacts across the entire food industry. From meat and poultry processors to potato sorting sheds, AI-driven inspection technologies present game-changing opportunities for companies to streamline quality control throughout their organization and improve accuracy, operational efficiency, and food safety.
Even still, many companies remain apprehensive about AI in food production. Integrating any new technology into a process comes with risks and challenges, but AI represents a significant organizational culture change. Additionally, with the rapid rise of AI companies in recent years, being skeptical about the reliability, accuracy, and security of these complex and expensive technologies is reasonable.
Regarding AI vision inspection systems in food production, there is one critical principle to keep in mind: AI applications are most successful when humans take the lead in training and maintaining the system.
The Differences Between Supervised and Unsupervised Learning
Food products are not manufactured to precisely engineered specifications like fabricated machine components. An acceptable tolerance for product appearance – size, shape, color, texture, volume, and others – is defined between the plant's operations and quality assurance teams. In most food operations, it is up to a human inspector standing in the line checking products for defects, out-of-spec features, or unwanted and potentially harmful foreign materials. This inspection method is inherently subjective and difficult to control at a single plant, let alone across an entire organization.
One of the central values of AI-driven vision inspection is its objectivity. Unlike a human operator, a well-trained AI system never has an “off-day” at the plant. It applies human-defined measurements to analyze products to an exact quality criterion. This method of AI training is called "supervised learning,” where the AI system is continually trained based on human inputs.
Supervised learning involves showing the AI some "good" samples (which could be tens of thousands of samples or more) and some "bad" samples, both labeled by a person or a team. Through this training method, a model is built based on specific algorithms. The AI system "learns" the acceptable criteria for a particular product feature or material. Each product model – including the ability to detect foreign materials – follows this process. Models evolve continuously as new samples are labeled and added into the training set for improved performance and reliability.
Supervised learning takes time and patience, but the effort will result in a trustworthy and accurate application.
The other method of AI training is called “unsupervised learning." Through this process, the AI application defines its own tolerances. Because AI constantly seeks data, unsupervised AI begins to make its own decisions on product quality, which can prompt unpredictable results.
The pitfalls of unsupervised learning are why companies should be wary of AI suppliers who do not demonstrate or offer consistent training of their AI models.
Where AI Applies to Solve Food Manufacturing Challenges Today
After picking from the field, fresh produce typically moves to a processing facility for sorting, sizing, and grading. Potatoes, for instance, are inspected and sorted on an extensive range of product features, including product shape, %green, cracks, bruises (old and new), sprouts, and many others. In a potato shed, an inspector usually makes these determinations and routes potatoes to their designated packing lanes in a fast-paced operating environment.
AI system measuring the "old bruise" percentage on a potato -- a product defect that can be costly if sorted for the wrong customer.KPM Analytics
AI systems with on-board cameras can scan incoming potatoes for their size and shape and sort out defective products with the help of automated rejection capabilities (e.g., robotic pickers, kicker paddles, and others). In more complex systems, a single potato can be placed into multiple lanes or drops to get that specific potato to the right customer. Again, the potato shed operator programs these tolerances with the assistance of their AI supplier, consistently refining these criteria over time.
In some cases, the AI system logs overall performance to help the operator make decisions for downstream processes. For instance, if there is a routinely high number of misses from an ejection kicker at a particular point in the process, it could signal a mechanical problem.
Foreign Material Detection & Regulatory Compliance
When the carcass arrives at the processing facility, undergoes its many cuts, and is either packed for the market or sent out for further processing, there are several moments in meat and poultry processing where unwanted foreign materials can enter. Anything from broken pieces of machinery to a rubber glove can cause catastrophic consequences. Considering the cost of a product recall – and the public relations nightmares that typically follow – meat and poultry processing companies using well-trained AI inspection technologies can instill greater protection to protect their customers and brand value.
As shown here, AI foreign material detection technology offers expansive capabilities to detect challenging materials missed by most inspection methods, such as cardboard on a chopped almond conveyor.KPM Analytics
While X-ray and metal detectors remain the industry’s most-used methods to detect foreign materials, both struggle to detect low-density or soft objects like paper, rubber, clear plastic, wood, and objects that may be of a similar color to the product. However, AI-driven inspection systems trained by operators allow companies to identify challenging free-flowing materials in products with mass variation (e.g., a meat or poultry trim line). Of course the limitation is that the object needs to be on the surface. Hence AI vision is complementary to X-Ray and metal detectors.
These systems can be programmed to instantly stop the processing line and let the operator remove the material, or they can be integrated with a rejection method to remove the material from the process automatically.
Additionally, in meat processing plants, AI-driven vision inspection can aid in the monitoring conditions of animals at intake. For instance, if chickens are meant to arrive to a processing plant alive, in good condition, or with a particular number of birds per crate, AI vision inspection can make these determinations in an instant. This helps companies keep their suppliers accountable while also providing proof of humane practices.
Production Process Control
AI-driven vision inspection technologies also aid in monitoring a production facility's overall health and performance. As an example, most high throughput baking operations follow core process steps like ingredient mixing, dough forming, and proofing. Then, after baking, the product cools before proceeding into sorting lanes for packaging.
The vision process control system monitors baguette dough for height, scoring depth, and other pre-baking quality features.KPM Analytics
Visual attributes in all these process steps can indicate the final product outcome. For example, a dough for a hamburger bun that did not proof to the ideal height before baking could produce a product that is too big or too small for the product packaging. When producing thousands of product units daily, having an inspector on the proofing line to identify minor variations like these is almost impossible to do accurately. However, a vision inspection system can identify these variations in height with superior accuracy and across 100% of products passing on the production line.
Similar applications exist for analyzing products after baking. For instance, long sheets of cracker dough pass through an oven with several individual heat sources above the product in a cracker production facility. With an AI-vision system trained to spot variations in product color, the operator can be alerted if a particular section of the baked cracker dough appears too dark or too light in color. These variations indicate the oven may need to be adjusted or serviced.
Human Intervention Vital to a Successful AI-Driven Inspection System
Despite what some AI inspection companies may advertise, no AI food inspection system exists today that arrives out of the box immediately, knowing a company’s unique product or production process. Continuous collaboration between the plant operators, quality assurance personnel, and a trustworthy AI vision inspection supplier is essential for driving a return on investment. By training and refining AI models, food processing companies can develop robust systems that amplify their quality assurance programs, reduce waste, improve accuracy, and redistribute labor where it is needed most.
Yuegang Zhao is the chief commercial officer at KPM Analytics.